Applications designed to predict mortality, often leveraging machine learning algorithms, analyze various factors to produce a projected lifespan. These factors might include demographics, health records, lifestyle choices, and genetic predispositions. The output is typically a probabilistic estimate of a person's remaining lifespan. Such applications are often viewed as an attempt to personalize life expectancy estimations, though their accuracy and ethical implications are subject to considerable discussion.
While offering potential insight into individual health trajectories, the importance and benefits of such applications remain controversial. Proponents suggest the ability to proactively address potential health concerns based on predicted outcomes, allowing for earlier interventions. Conversely, concerns regarding the accuracy of predictions, the potential for misuse of data, and the ethical implications of using such tools for life planning are significant. The historical context of attempts to predict mortality includes actuarial tables and other demographic modeling; these earlier methods laid the foundation for current computational models but lacked the granularity of contemporary algorithms.
The subsequent discussion will delve into specific examples of these prediction systems, explore their methodological underpinnings, and examine the crucial ethical considerations surrounding their utilization.
AI Death Prediction Calculator App
Applications designed to predict mortality, leveraging algorithms, raise complex ethical and practical concerns. Understanding the key aspects of such tools is crucial.
- Data Input
- Algorithmic Model
- Prediction Accuracy
- Ethical Implications
- Privacy Concerns
- Misinterpretation Potential
- Public Perception
- User Interface Design
Data input dictates the reliability of predictions; complex models enhance accuracy but introduce potential bias. Accuracy benchmarks and potential for misinterpretation need thorough examination. Privacy concerns and ethical implications regarding sensitive data are paramount. Public perception can influence adoption and societal acceptance. User experience in tools like these impacts trust and practical use. Design considerations should prioritize transparency and clarity to avoid misinterpretation. Ultimately, the success and responsible use of such tools necessitate careful consideration of all aspects, with a strong emphasis on transparency and ethical use. For example, misinterpretation of a probabilistic prediction could lead to unnecessary stress or inaction. Thorough user interface design plays a crucial role in mitigating these risks by ensuring clear and understandable presentation of predictions.
1. Data Input
The accuracy and reliability of predictions generated by a mortality prediction application are directly contingent upon the quality and completeness of input data. Data input serves as the foundation upon which the predictive model operates, and the robustness of this foundation significantly influences the validity of the resulting estimations.
- Demographic Data
Age, gender, and ethnicity are fundamental factors affecting life expectancy. Incorporating these data points allows the model to establish baseline comparisons and identify potential risk factors. However, reliance solely on these broad categories can lead to inaccurate estimations, especially when considering varying socioeconomic and lifestyle factors. Examples include differing access to quality healthcare or prevalence of specific diseases within particular demographic groups.
- Health Records
Comprehensive medical history, including diagnoses, treatment plans, and medication records, provides crucial insights into individual health trajectories. Data on chronic conditions, previous hospitalizations, and surgical interventions offers a more detailed profile, informing a more nuanced prediction. However, data quality and potential inconsistencies or missing information within patient records remain challenges. The completeness and consistency of health records significantly affect predictive accuracy, and limitations can generate misleading estimates.
- Lifestyle Factors
Data on lifestyle choices, such as diet, exercise habits, smoking history, and alcohol consumption, contribute to understanding individual risk profiles. These factors, alongside genetics and environmental influences, contribute substantially to overall health and longevity. However, self-reported lifestyle data may lack accuracy or be subject to recall bias, impacting the reliability of predictions. Discrepancies between reported and actual behaviors create challenges for the model's predictive capabilities. Furthermore, the influence of social determinants on health might be challenging to quantify within the input data.
- Genetic Information
Genetic predisposition to various diseases offers insights into potential health risks. However, ethical considerations and data privacy must be paramount. The potential for misinterpretation of genetic information and the complex interplay of genes with environmental factors require careful evaluation. Further research is needed to determine the optimal way to incorporate genetic data while preserving privacy and upholding ethical standards.
Ultimately, the quality and comprehensiveness of input data are critical for the reliability of predictions generated by these applications. Addressing potential limitations and biases in data input, such as data quality, completeness, and potential inaccuracies in self-reporting, is essential to developing a robust and ethically sound mortality prediction system.
2. Algorithmic Model
The algorithmic model underlying a mortality prediction application dictates how input data is processed and transformed into predictions. Its structure and complexity significantly impact the accuracy and reliability of the estimations. The model's effectiveness hinges on the ability to identify patterns and correlations within the data to project future health trajectories. This section explores crucial components of these models.
- Statistical Modeling Techniques
Applications frequently employ statistical methods like survival analysis, regression models, and machine learning algorithms. Survival analysis models track the time until an event (death) occurs, while regression models examine the relationship between various risk factors and life expectancy. Machine learning approaches, including neural networks, can identify intricate patterns in data that may be missed by traditional methods. Each technique has strengths and limitations, affecting the model's accuracy. For instance, linear regression might struggle with non-linear relationships in the data, whereas neural networks can potentially capture these complex patterns but might be more prone to overfitting or require extensive data.
- Feature Engineering
Transforming raw input data into relevant features crucial for the model's prediction accuracy is vital. Feature engineering processes can include selecting relevant variables, generating derived features, and standardizing or scaling the data. The process can range from simple calculations like age normalization to complex techniques using existing medical research or patient behavior patterns. Effective feature engineering is essential, as poor feature selection can lead to inaccurate or biased predictions. A model might interpret, for example, a patient's history of high cholesterol as a minor factor because other, more impactful variables are not included.
- Model Validation and Calibration
Rigorous validation and calibration of the model are essential to ensure its reliability. Techniques include cross-validation and hold-out sets to evaluate the model's performance on unseen data. Calibration involves adjusting the model's predictions to ensure they align with observed mortality rates within specific groups, thereby enhancing accuracy. This validation and calibration process aims to prevent overfitting and guarantee reasonable accuracy on new, independent data sets. Overfitting occurs when the model learns too much from the training data, which can give overly confident but inaccurate results.
- Bias and Fairness Considerations
Algorithmic models can inherit and amplify biases present in the input data, potentially leading to discriminatory outcomes. Bias mitigation strategies, such as data preprocessing techniques and model adjustments, are essential to develop fair and equitable mortality prediction applications. A model trained on biased data might systematically underestimate mortality risks for certain demographic groups, leading to potentially harmful outcomes. Ensuring fairness and equity in these models is a critical consideration, as inaccuracies can have serious consequences.
Ultimately, the algorithmic model's design and implementation significantly impact the predictive capabilities of a mortality prediction application. Careful consideration of statistical methods, feature engineering, and validation techniques is crucial for producing reliable and trustworthy estimations, while also addressing and mitigating the risk of bias. A robust model must stand up to rigorous scrutiny, ensuring responsible use of the technology.
3. Prediction Accuracy
The accuracy of mortality predictions generated by applications is a critical consideration. Inaccurate estimations could lead to misinformed decisions regarding health management, financial planning, and end-of-life care. This section examines the factors influencing prediction accuracy, emphasizing the limitations and potential pitfalls of these applications.
- Data Quality and Completeness
The quality and comprehensiveness of input data directly impact the accuracy of predictions. Inaccurate or incomplete data introduce errors and limitations in the analysis. For instance, if a patient's medical history is missing key diagnoses or treatments, the model's ability to assess future health risks will be compromised. The availability of reliable and comprehensive data is a significant challenge for accurately predicting mortality.
- Model Complexity and Underlying Assumptions
Complex models, while potentially capturing intricate relationships within data, may also rely on assumptions that limit accuracy and generalizability. The models' reliance on specific statistical methods or machine learning algorithms, as well as the limitations of these methods themselves, can introduce biases and uncertainties in the predictions. For example, a model heavily relying on historical trends might not accurately account for rapid advancements in medical science or evolving lifestyle patterns. These limitations can lead to inaccurate projections of lifespan.
- External Factors and Future Events
Unexpected external factors and future events, such as unforeseen health crises or breakthroughs in medical treatments, can significantly impact mortality predictions. The inherent variability in individual health trajectories and external influences means models can sometimes struggle to adequately reflect the future. Predicting complex, unpredictable circumstances, such as outbreaks of contagious diseases, can be particularly problematic. The unpredictability of future events poses a fundamental limitation on the accuracy of these applications.
- Bias in Data and Model Training
Existing biases within the input data or in the model's training can propagate inaccuracies in the predictions. Model biases might lead to skewed representations of mortality risks for certain demographic groups. If the training data reflects existing societal inequalities or disparities in healthcare access, the resulting model may amplify these inequalities, producing inaccurate predictions for underrepresented populations. Understanding and mitigating potential biases is vital for the equitable deployment of these applications.
Ultimately, the accuracy of mortality prediction applications is a multifaceted issue. Recognizing the limitations imposed by data quality, model assumptions, external factors, and potential bias is crucial. Developing transparent and robust methodologies for evaluating and reporting predictive accuracy is essential to ensure that such tools are utilized responsibly and effectively. The ongoing refinement of these tools requires careful evaluation of their predictive performance in diverse and representative populations.
4. Ethical Implications
Applications designed to predict mortality raise profound ethical concerns. The potential for misuse, the impact on individual autonomy, and the implications for societal values necessitate careful consideration. Transparency, accountability, and equitable access are crucial in navigating these complexities. Unintended consequences, stemming from biases within data or model assumptions, must be anticipated and mitigated.
- Data Privacy and Security
The collection, storage, and use of sensitive personal data are central concerns. Protecting patient confidentiality and preventing unauthorized access or misuse are paramount. The potential for data breaches or inappropriate sharing of information necessitates strong security protocols and robust data governance frameworks. For example, a data breach could expose individuals' predicted mortality risks, leading to potential discrimination or anxieties. The data's secure handling is therefore essential in preventing exploitation and ensuring user trust.
- Bias and Discrimination
Algorithmic models can inherit and amplify biases present in training data, potentially leading to discriminatory outcomes. These biases can arise from various sources, including socioeconomic disparities, historical inequalities in healthcare access, and implicit prejudices within datasets. For example, if the training data disproportionately reflects the health experiences of a particular demographic group, the model may incorrectly predict mortality risk for others. Addressing potential biases in the data collection process and model development is paramount for ensuring fair and equitable outcomes.
- Impact on Individual Autonomy and Decision-Making
Predictive models can influence individual choices regarding healthcare, lifestyle, and financial planning. The potential for such tools to alter patients' autonomy and lead to anxieties or undue pressure warrants careful consideration. Individuals might feel pressured to adopt specific lifestyles or treatments based on predicted outcomes, potentially compromising their self-determination. Transparency regarding the limitations and potential uncertainties of the predictions is essential to ensure informed decision-making.
- Societal Implications and Access to Resources
Unequal access to these predictive tools raises concerns about equity and resource allocation. If such applications are primarily accessible to wealthier individuals or specific demographics, this could exacerbate existing health disparities. Ensuring equitable access to these tools and the supporting resources is critical to prevent the perpetuation of health inequities. For example, lack of access to high-quality healthcare could make accurate predictions difficult and further marginalize already disadvantaged groups.
These ethical concerns underscore the need for careful consideration and proactive measures to ensure responsible development and deployment of mortality prediction applications. Ongoing dialogue between researchers, policymakers, clinicians, and the public is essential for navigating the complex ethical landscape presented by these innovative tools. Robust oversight mechanisms and ethical guidelines must be in place to mitigate potential harms and maximize societal benefits.
5. Privacy Concerns
The development and deployment of AI-powered mortality prediction applications raise significant privacy concerns. These applications necessitate the collection and analysis of sensitive personal data, including medical history, lifestyle choices, and genetic information. This data, if mishandled or accessed inappropriately, can have devastating consequences for individuals. The potential for misuse, whether intentional or accidental, necessitates stringent safeguards and ethical considerations. The interconnectedness of privacy and predictive applications demands attention.
Consider a scenario where a user's data is compromised. Exposing detailed health predictions and potential life expectancies could lead to discrimination in insurance or employment. Privacy breaches could further exacerbate existing societal inequalities. For example, individuals with predispositions to specific illnesses might face higher premiums or denied coverage, based solely on predicted outcomes. Moreover, unauthorized access to this data could compromise patient autonomy and engender unwarranted anxiety. The potential for misinterpretation or misuse of these predictions underscores the necessity for strong data protection measures. The very premise of these applications necessitates a robust framework for data security and ethical usage.
A crucial aspect of this interplay lies in the potential for data misuse. Detailed predictions of mortality, if exploited, can lead to discriminatory practices and compromise individual autonomy. Ensuring data security and maintaining public trust are paramount. This understanding necessitates a focus on transparent data handling, explicit consent processes, and stringent security measures. These applications must operate within a robust regulatory framework that safeguards sensitive personal information. The development and deployment of such applications must be accompanied by a thorough assessment of privacy implications and proactive measures to prevent misuse. This necessitates a critical consideration of privacy as a critical component of the application, requiring measures for protecting individuals from potential harm.
6. Misinterpretation Potential
Mortality prediction applications, often employing complex algorithms, offer potential for misinterpretation. The intricacy of the underlying models, coupled with the sensitivity of the predicted outcomes, can lead to inaccurate conclusions and subsequent detrimental actions. Users might misinterpret probabilistic estimations, leading to inappropriate decisions regarding healthcare choices, financial planning, or lifestyle adjustments. A predicted higher likelihood of mortality might trigger unwarranted anxiety or avoidance of potentially beneficial medical interventions, while a lower prediction might lead to complacency. Consequently, understanding this misinterpretation potential is crucial to responsible application and to mitigating potential harms.
Real-world examples illustrate the significance of this issue. A user might interpret a predicted higher likelihood of mortality within a certain timeframe as an absolute certainty, leading to significant distress and possibly avoidance of necessary medical care. Conversely, a low predicted risk might lead individuals to postpone preventive measures or neglect appropriate health management. Furthermore, the presentation of probabilistic predictions, often expressed as percentages or ranges, might be misconstrued as definitive statements, further compounding the risk of misinterpretation. The complexity of the underlying calculations, while intended to enhance prediction accuracy, can also obscure the inherent probabilistic nature of the outputs, thereby potentially encouraging misinterpretations by users lacking a comprehensive understanding of the methodology.
The practical significance of understanding misinterpretation potential lies in the development of user-friendly interfaces that emphasize clarity and transparency. Clearly presenting the probabilistic nature of the predictions is essential. Displaying predictions alongside explanations of the methodology and associated uncertainties can enhance comprehension and reduce misinterpretations. Moreover, integrated tools providing additional contextual informationsuch as explanations of the risk factors considered and the potential impact of lifestyle adjustmentsare vital for promoting informed decision-making. Education campaigns focused on the probabilistic nature of these predictions and the importance of seeking professional medical advice can also play a critical role in reducing the risks associated with misinterpretations. Ultimately, emphasizing the inherent limitations of these tools is paramount to preventing harm.
7. Public Perception
Public perception significantly influences the acceptance, adoption, and impact of AI-powered mortality prediction applications. Public understanding and trust are critical factors in determining the successful integration and responsible use of these tools. This section explores facets of public perception pertinent to these applications.
- Trust and Credibility
Public trust in the accuracy and reliability of AI predictions plays a pivotal role. Applications must demonstrate trustworthiness and demonstrate high accuracy rates through rigorous validation and clear communication. If public trust is eroded due to inaccurate predictions or perceived biases, adoption may falter. Misinterpretations or misuse of the technology can also negatively impact public trust. Public perception of similar technological advancements (e.g., AI in healthcare diagnoses) can inform public reaction to these mortality prediction applications.
- Fear and Anxiety
The prospect of knowing one's predicted mortality can evoke fear and anxiety. Public perception might be influenced by concerns about the psychological impact of such predictions. Understanding potential anxieties and developing tools to address concerns associated with receiving predictions are crucial. For instance, the way prediction results are presented (i.e., probabilities vs. absolutes) can influence public reaction and perception.
- Ethical Concerns and Societal Impact
Ethical considerations related to data privacy, potential bias in predictions, and the impact on societal values can shape public opinion. Public discussions addressing these ethical implications are necessary to promote responsible use and foster trust in these applications. Transparency and clear communication regarding the limitations of these prediction tools are crucial to manage public concerns effectively.
- Accessibility and Equity
Public perception regarding accessibility and equitable access to these prediction applications can influence acceptance and potential societal impact. Disparities in access to technology or financial resources can lead to concerns about social justice issues. If public perception suggests limited access based on socioeconomic factors, this can hinder adoption and potentially exacerbate existing health inequalities. Strategies for equitable access to these tools need to be carefully considered.
Ultimately, public perception of AI-powered mortality prediction applications is a complex interplay of trust, fear, ethical concerns, and considerations of accessibility. Successfully navigating this landscape requires thoughtful communication, addressing ethical issues proactively, and ensuring equitable access. A robust and transparent framework for the development and deployment of such applications can significantly impact public perception, fostering trust and promoting responsible usage. Understanding the nuanced aspects of public perception is essential for the successful integration of these tools into society.
8. User Interface Design
User interface (UI) design plays a critical role in the efficacy and ethical deployment of AI death prediction calculator applications. A well-designed UI can significantly impact user experience, fostering trust and enabling informed decision-making. Conversely, a poorly designed UI can contribute to misinterpretation, anxiety, and potentially harmful actions. Clear and concise presentation of complex probabilistic data is paramount to avoid misleading or distressing users.
The UI must effectively communicate the inherent uncertainties and limitations of the predictions. Presenting data in a format that clearly distinguishes probability from certainty is crucial. Visualizations, such as graphs and charts, can effectively convey the probabilistic nature of predictions. Visual representations of risk factors contributing to the prediction enhance transparency and aid users in understanding the model's considerations. Examples of effective UI design include clear labels, intuitive navigation, and user-controllable visualization options. Furthermore, incorporating elements such as explanations of the model's workings, potential limitations, and guidance on interpreting the results can enhance comprehension and mitigate misinterpretations.
Practical applications of this understanding involve designing interfaces that prioritize transparency and clarity. Real-world examples include applications that present predictions not as absolute values but as ranges, acknowledging the inherent variability in individual health trajectories. Additionally, incorporating clear disclaimers regarding the limitations of the application and suggestions to consult medical professionals can mitigate potential risks. Ultimately, a user-centered design approach, ensuring easy navigation and readily accessible information, is critical for effective and responsible use of such applications. By addressing user needs and facilitating comprehension, UI design directly impacts the reliability and ethical application of these predictive tools, minimizing potential harm arising from misinterpretation or misapplication of the presented data.
Frequently Asked Questions about AI Death Prediction Calculator Apps
These frequently asked questions address common concerns and misconceptions surrounding applications designed to predict mortality. The following Q&As aim to provide clarity and context, emphasizing the limitations and ethical considerations associated with such tools.
Question 1: How accurate are these predictions?
Accuracy varies significantly based on the quality and completeness of input data, the complexity of the underlying algorithm, and external factors impacting individual health trajectories. While advanced algorithms might identify patterns and correlations, unforeseen health events or changes in lifestyle can affect the reliability of predictions. No application can guarantee perfect accuracy for any individual.
Question 2: What data does the application need?
Applications typically require demographic information, health records (including diagnoses, treatments, and medications), lifestyle factors (such as diet, exercise, and smoking history), and potentially genetic information. The specific data requirements vary between applications and models.
Question 3: Are these predictions reliable for personal decisions?
Mortality prediction applications offer insights but should not be solely relied upon for critical personal decisions. These predictions are estimations based on available data, and unforeseen factors can impact individual health. Professional medical advice and careful consideration of all available information are essential.
Question 4: What are the ethical concerns surrounding these applications?
Ethical concerns include data privacy and security, the potential for bias in predictions, and the impact on individual autonomy. Discrimination based on predictions, concerns about psychological effects, and the responsible use of sensitive data require careful consideration and robust safeguards.
Question 5: How can I ensure my data is secure?
Choosing applications with strong data security protocols, understanding the data handling policies of the platform, and exercising caution regarding data sharing are crucial. Transparency in data handling practices is essential for user trust and security. Users should actively manage their data access and settings within the applications.
Question 6: How can I use these applications effectively?
These tools should be viewed as supporting resources rather than definitive guides for decision-making. Consult with medical professionals to interpret predictions within the context of individual health situations. An informed understanding of the tool's limitations and transparent communication of results are key for responsible use.
In summary, AI-powered mortality prediction applications can provide insights but not definitive answers. The tools require careful consideration, transparency, and responsible interpretation to avoid misapplication and potential harm. These considerations are paramount to effective and ethical use.
The subsequent section will delve into the technical aspects of these applications, examining the underlying algorithms and methodologies used in mortality prediction.
Tips for Using AI Mortality Prediction Applications
Applications designed to predict mortality offer potential insights, but responsible use requires careful consideration. These tips aim to guide users toward effective and ethical application of these tools.
Tip 1: Prioritize Transparency and Understanding. Seek clarity regarding the methods and data employed by the application. Understanding the limitations and potential biases inherent in the model is crucial. Review the application's data privacy policies and data security measures. Comprehending how the model operates and how external factors can affect predictions is essential for responsible use.
Tip 2: Acknowledge Probabilistic Nature of Predictions. Recognize that predictions represent probabilities, not certainties. Avoid interpreting percentages as absolute guarantees. Understand that predictions are estimations based on current knowledge and data; unforeseen circumstances and individual variability can alter outcomes.
Tip 3: Consult Healthcare Professionals. Interpret predictions in conjunction with medical advice. Discuss predictions with healthcare providers to understand their implications for individual health plans and to address any anxieties or concerns. Professional guidance is critical for navigating the complexities of such estimations.
Tip 4: Avoid Overreliance on Predictions. These applications are tools to inform decision-making, not dictate it. Avoid making crucial decisions based solely on predictions. View estimations as one factor among many in comprehensive health planning.
Tip 5: Evaluate Data Sources and Model Reliability. Critically assess the data used in the application's algorithms. Seek applications using validated methodologies and reputable data sources. Look for independent verification of the application's accuracy and transparency in the model's construction.
Tip 6: Understand Limitations of Prediction Accuracy. Be mindful that predictions are not infallible. External factors and future events can influence outcomes, potentially affecting prediction accuracy. These applications serve as supplemental information and should not replace expert clinical judgment.
Tip 7: Focus on Informed Decision-Making. Use insights from the application to supplement existing knowledge. Employ predictions as a tool to inform conversations with healthcare providers. Prioritize a balanced and holistic approach to healthcare planning, integrating these insights with expert medical advice and personal values.
Tip 8: Address Potential Emotional Impacts. Recognize that receiving predictions concerning mortality can evoke various emotions. Seek support systems, such as family and friends, or mental health professionals, if necessary. Maintaining emotional well-being is crucial when considering estimations of mortality risk.
By following these guidelines, users can leverage AI mortality prediction applications responsibly, ensuring that insights contribute positively to informed decision-making, rather than exacerbating anxieties or leading to potentially harmful actions.
The continued development and responsible application of these technologies demand ongoing dialogue and ethical considerations. Ultimately, a proactive approach emphasizing understanding, consultation, and critical evaluation is crucial for maximizing the potential benefits while mitigating the associated risks.
Conclusion
The exploration of AI death prediction calculator apps reveals a complex interplay of potential benefits and significant ethical concerns. Accuracy hinges critically on the quality and completeness of input data, the robustness of the underlying algorithms, and the inherent limitations of predicting future events. While these tools might offer insights into potential health trajectories, the probabilistic nature of the predictions necessitates cautious interpretation and underscores the importance of professional medical guidance. Ethical considerations, including data privacy, potential bias in algorithms, and the impact on individual autonomy, demand stringent safeguards and transparent practices. The potential for misinterpretation and undue anxiety warrants careful design of user interfaces and accompanying educational materials. Furthermore, ensuring equitable access and addressing potential societal implications are essential for responsible implementation.
Moving forward, responsible development and deployment of AI death prediction calculator apps require a collaborative effort involving researchers, policymakers, ethicists, and the public. Robust regulatory frameworks, transparent communication, and ongoing evaluation are indispensable to maximize the potential benefits while mitigating potential harms. The long-term impact of these tools depends on the commitment to ethical considerations, rigorous validation, and user-centered design. The development and use of such applications must prioritize the well-being and autonomy of individuals while respecting the complexity of human health and life's unpredictable nature.
Article Recommendations
- East Multnomah Soil And Water Conservation District
- Short Positive Quotes About Life Challenges
- Rodney Alcala On Dating Game Video

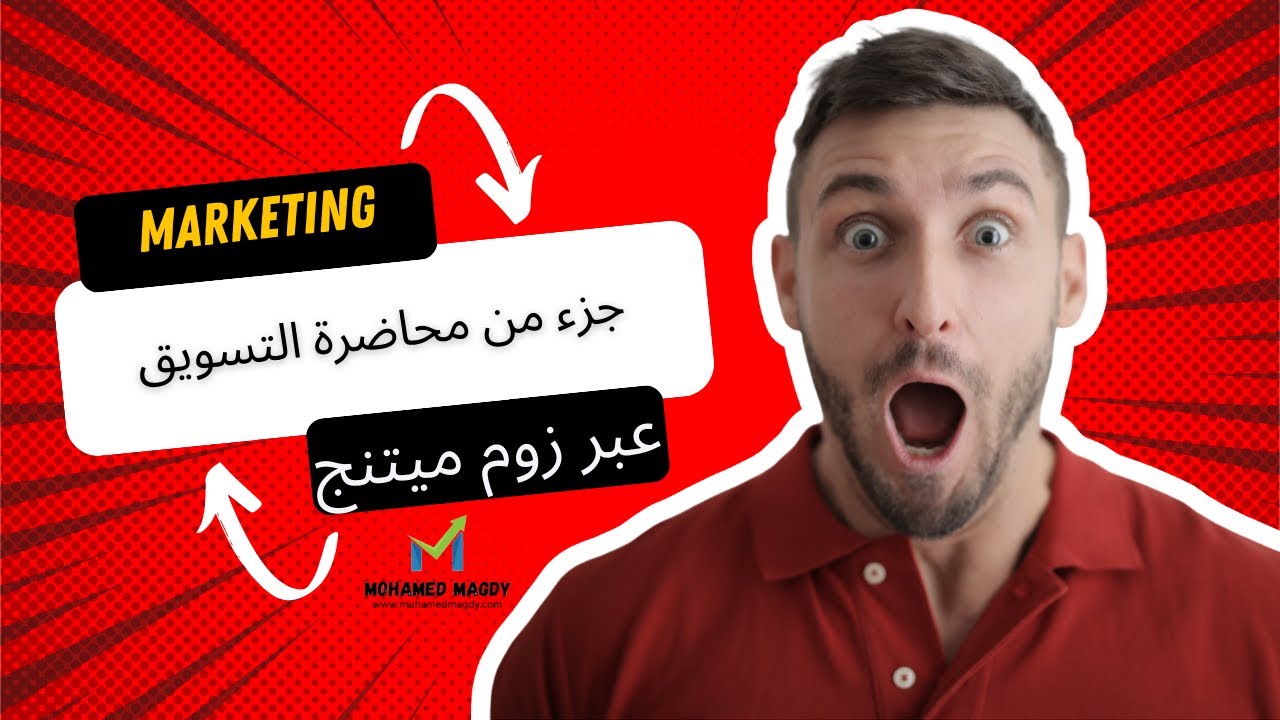
